The Eric and Wendy Schmidt AI in Science Postdoctoral Fellowship
The Eric and Wendy Schmidt AI in Science Postdoctoral Fellowship, a program of Schmidt Sciences, is a new international initiative that seeks to change how science is done by accelerating the incorporation of AI techniques into the natural sciences, engineering, and mathematical science (STEM), providing access to AI tools and training to the sharpest minds on the frontlines of scientific innovation.
The program aims to create great science; an acceleration of the substantive use of AI in imagining, planning, executing, and supporting scientific research around the world; and the building of a global cohort of scientific leaders with a grounding in the application of AI in their fields.
Fellows will have the opportunity to pursue original research on significant questions in AI and science. They will form a cohort of top scholars across the natural sciences engaging in joint training and research activities. Fellows will be provided with a unique, cross-campus ecosystem of training in AI methods necessary for conducting their research. They will receive a competitive salary and benefits, generous travel allowances, and the opportunity to collaborate with partners worldwide.
Eligibility Criteria
- Applicants must be within 3 years of having obtained PhD degree or equivalent degree in non-computer science areas.
- Excellent academic records and research potential.
- Applicant is required to submit a thoughtful and realistic inter-disciplinary research proposal. Only research on applying AI techniques to engineering, natural sciences and mathematical sciences will be supported.
- Applicants are required to identify a potential project mentor (i.e. NTU Faculty), who can provide access to valuable academic and career guidance.
Fellowship Terms and Conditions
- The fellowship will be tenable for up to two (2) years with an attractive remuneration package.
- Awardees will be required to take at least one AI module every year during the fellowship.
- Awardees are required to submit an annual progress report to the University and Schmidt Futures, stating their progress, results and contributions.
- Awardees are required to attend conferences/workshops/seminars organized by the host University and Schmidt Futures to present their research and seek collaborations.
Application Process
- The Eric and Wendy Schmidt AI in Science Postdoctoral Fellowship 2024 Call for Application is currently OPEN.
- Candidates interested to apply for the scheme are required to contact their potential project mentor (i.e. NTU Faculty) and work with the potential mentor in submitting their application package. Please check with the project mentor for internal deadline.
- Each applicant is required to submit the complete application form (Download Template) with the following supporting documents:
- 2-page research proposal (Section 7 (i) on application form)
- Comprehensive CV (with full publication list)
- Official Doctorate transcript or Degree Certificate in English
- Two reference letters (one must be from PhD Supervisor)
- Top 3 publications (in PDF) and any other supporting documents (scanned copy)
Queries can be sent to Dr Yao Wei ([email protected])
Awardees
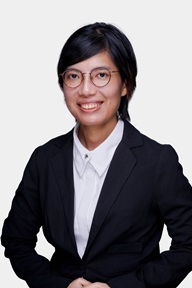
Lai Kei Onn
Host School : LKCMedicine
Email: [email protected]
LinkedIn: https://www.linkedin.com/in/kei-onn-lai-phd-70118b202/
Github: https://github.com/klai001
Kei Onn obtained her PhD from the Nanyang Technological University in August 2023. During her PhD, she was mentored by Dr Anna M. Barron (Neurobiology- main mentor) and Dr Sarah R. Langley (bioinformatics- co-mentor). Her integrated doctoral training
in experimental and computational approaches sparked her interest in the meaningful translation of computational techniques to investigate the molecular pathophysiology of neurodegenerative diseases. Following her doctoral training, her current work
is centered on understanding cognitive resilience in Alzheimer’s Disease. This work intends to employ machine learning techniques that will be evaluated on their suitability not only based on the data, but also aims to be grounded on biological truths.
- Machine learning techniques in multi-omics
- Graph theoretical approaches for network biology
Project Title: Drug repurposing for Alzheimer’s Disease based on molecular networks of amyloid positive but cognitive normal individuals.
Project Abstract: Prior to symptoms onset, beta-amyloid is the initiator of the Alzheimer’s Disease (AD) neurodegenerative cascade. Intriguingly, beta amyloid accumulation (amyloid positive) in the brain of cognitive normal individuals have been reported. However, it is unclear why some amyloid positive individuals develop cognitive impairment, while others are spared. Herein, I hypothesize that cognitively normal, beta amyloid-bearing individuals may harbour protective mechanisms against the beta-amyloid initiated neurodegeneration cascade. I will leverage on machine learning techniques to provide molecular insights on the cognitive resilient population as well as identifying other endotypes of AD cohort. Molecular targets identified are intended to assist in drug repurposing for AD therapeutics.
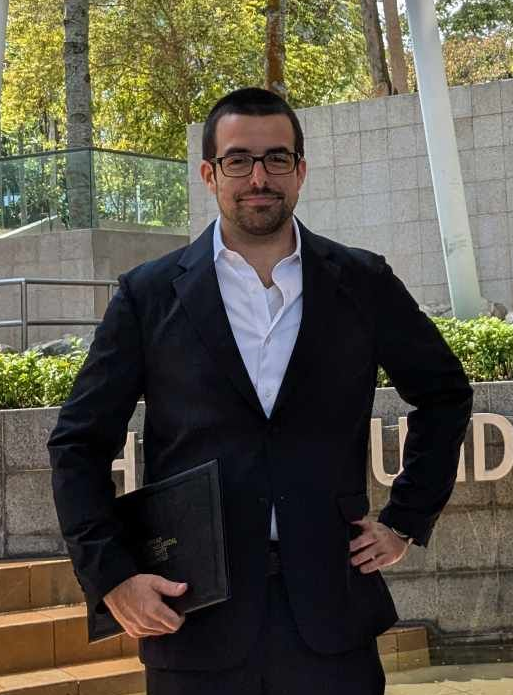
Benjamin Lebeau
Host School : SBS
Email: [email protected]
Benjamin was born in the francophone town of Joliette, Québec, Canada. He studied cell biology, epigenetics and cancer during my PhD studies at McGill University, in Michael Witcher’s lab. He is now working on epigenetic, 3D chromatin structure
and artificial intelligence at NTU, in Melissa Fullwood’s lab
- Study the interplay between 3D chromatin structures, gene expression and the epigenetic landscape
- Target aberrant 3D chromatin structures associated with cancer to develop new treatments
- Use Artificial Intelligence to predict 3D chromatin structures at the single cell level
Project Title: Enhancing Single-Cell Multiomics with DisMuCh: An AI-Integrated Direct Sequencing Method.
Project Abstract: To properly regulate gene expression, DNA is hierarchically organized within the nucleus of each cell. Alteration of this 3D genome organization is often observed in tumors and has been shown to promote cancer progression. As tumors can be highly heterogeneous, with tumor sub-populations progressing toward distinct chromatin organization and gene expression patterns, investigating 3D genome organization at the single-cell level could improve the specificity of epigenetic therapies. However, single-cell investigation of chromatin conformation is limited by the low efficiency of current methods. Therefore, we hypothesize that by maximizing the epigenetic information we detect from each cell, we can utilize Artificial Intelligence (AI) to accurately predict 3D genome organization at the single-cell level. To do so, we are developing Direct Sequencing of Multiomic Chromatin information (DiSMuCh). DiSMuCh protocols yield a series of proximally ligated and cell identity-barcoded hybrid fragments of kethoxal-treated and natively methylated DNA and RNA, which are directly sequenced using Nanopore. DiSMuCh yield captures, at the single-cell level: 3D chromatin organization involving DNA-DNA, RNA-DNA, or RNA-RNA interactions, DNA/RNA base modification, and actively transcribed regions of the genome, maximizing the information gained on each read. Following sequencing, explainable AI will integrate this multiomics information captured to accurately predict the 3D genome organization at the single-cell level and output which epigenetic factors are driving the prediction. Finally, the resulting high-resolution single-cell representation of 3D chromatin structure and multiomics information will enable a clear and accurate study of driving events of tumor evolution, which could be targeted for safer and more efficient treatment.
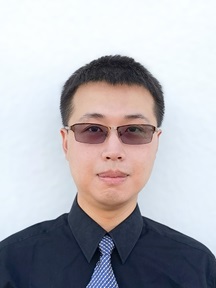
Ning Zepeng
Host School : EEE
Email: [email protected]
Zepeng Ning received his B.S. degree in electronic information science and technology and his M.S. degree in control science and engineering, both from the Harbin Institute of Technology, China, in 2014 and 2016, respectively. He received his Ph.D. degree
in control science and engineering from Harbin Institute of Technology in 2021, during which he was also a joint Ph.D. student with the Department of Chemical and Biomolecular Engineering at the University of California, Berkeley, CA, USA. He held
several research positions, including a Research Associate with the Department of Mechanical Engineering at The University of Hong Kong, China, a Research Fellow with the School of Chemistry, Chemical Engineering and Biotechnology at Nanyang Technological
University, Singapore, and a Research Fellow with the School of Electrical and Electronic Engineering at Nanyang Technological University, Singapore. He is currently a Schmidt AI in Science Fellow at Nanyang Technological University, Singapore.
- Carbon capture processes
- Chemical and biomolecular system regulation
- Learning-based control
- Safe reinforcement learning
- Stochastic switching systems and probabilistic decision
- System modeling and optimization
Project Title: Safe Reinforcement Learning for Autonomous Model Predictive Control of CO2 Capture Based on Semi-Markov Decision Process.
Project Abstract: The research project focuses on developing safe reinforcement learning (RL) for autonomous model predictive control in carbon capture in a semi-Markov decision process framework. This interdisciplinary effort aims to advance carbon neutrality by innovating smart process operations in chemical engineering and automatic control. Safe RL technique is employed to manage uncertainties and ensure operational safety in dynamic environments, which facilitates robust, autonomous model predictive control strategies for carbon capture processes. By integrating safe RL with semi-Markov decision processes, the project seeks to create new theories, algorithms, and systematic solutions for system modeling and smart decision-making. This approach is expected to inspire global research in AI and related fields, potentially revolutionizing carbon capture technologies and promoting smart manufacturing and digital transformation. Ultimately, the incorporation of these AI techniques is leveraged to enhance the efficiency and safety of carbon capture systems, which contributes to smarter and more sustainable industrial processes.

Vo Dat Nguyen
Host School : CCEB
Email: [email protected]
Google Scholar: https://scholar.google.com/citations?user=6DpchCQAAAAJ&hl=en
Dr. Nguyen’s research interests encompass mathematical modeling, process design, process optimization, and machine learning in energy, environmental, and chemical processes. During his research career, Dr. Nguyen has authored more than 20 scientific
papers, with half of them published in prestigious journals (Chem. Eng., Appl. Energy, Energy Convers. Manag., Renew. Sustain. Energy Rev.). His contributions have earned his numerous fellowships and awards, including the Honor Prize for Undergraduate
Student (Vietnam, 2013), BK21 Excellent Researcher (Korea, 2020), the BK21 Postdoctoral Fellowship (Korea, 2022), and the Eric and Wendy Schmidt in Science Fellowship (Singapore, 2024).
Research Interests:
- Process Systems Engineering
- Energy Systems Engineering
- CO2 capture and utilization
- H2 production
Project Title: Machine Learning assisted Design and Operation of a CO2 capture and Conversion Process using Renewable Energy.
Project Abstract: This project suggests a unified framework and associated approaches for employing machine learning (ML) to assist in designing and operating an integrated CO2 capture and conversion (i3C) process using renewable energy such as solar and wind. The primary objective encompasses ML-assisted design and operation, focusing on optimizing the i3C process using renewable energy and addressing energy fluctuation inherent in such systems. ML-assisted design aims to identify the optimal operating and design parameters for the i3C process, leveraging renewable energy inputs. By utilizing deep neural networks (DNN), a surrogate model for the i3C process is developed, facilitating rapid evaluation and optimization. Additionally, a data-driven model is employed to forecast renewable energy availability, providing crucial insights for the design optimization process. The ML-assisted operation tackles the dynamic optimization of parallel energy buffer modules to mitigate fluctuation in renewable energy sources. This involves utilizing recurrent neural networks RNN) and reinforcement learning (RL) to address the inherent variability of solar and wind energy inputs. By leveraging ML techniques, this project aims to significantly enhance the efficiency, reliability, and sustainability of the i3C process integrated with renewable energy sources.
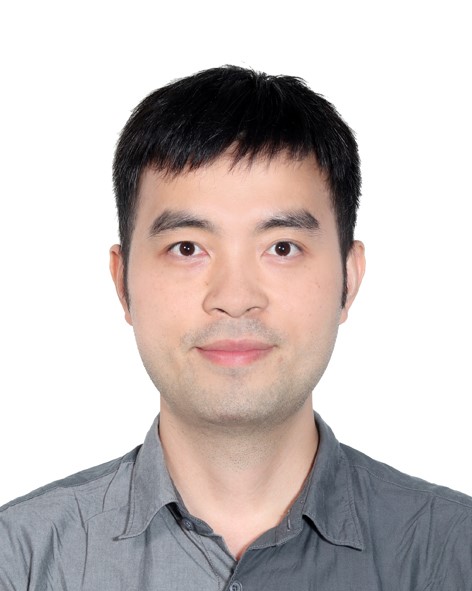
Yang Chengran
Host School : SPMS
Email: [email protected]
Dr. Chengran Yang earned his Bachelor's degree in Mathematics from Zhejiang University and his Ph.D. in Physics from Nanyang Technological University. He is currently the Eric and Wendy Schmidt AI in Science Postdoctoral Fellow at the School of Physical and Mathematical Sciences, Nanyang Technological University. His research spans tensor networks, quantum information, quantum algorithms, machine learning, quantum machine learning, quantum stochastic modelling and quantum finance, pushing the boundaries of applications in artificial intelligence and quantum technology.
Research Interests:
- tensor networks
- quantum information,
- quantum algorithms,
- machine learning,
- quantum machine learning,
- Quantum stochastic modelling,
- quantum finance
Project: Machine-Learning-Enhanced Quantum Stochastic Modelling
Project Abstract: Complex stochastic processes are at the heart of various natural and social phenomena like financial markets, weather patterns, and language processes. Although large models deliver impressive results, they demand substantial memory. Quantum models, on the other hand, harness distinct attributes absent in their classical counterparts, offering significant potential for memory reduction in simulating stochastic processes. This proposal seeks to leverage machine learning techniques to devise algorithms that identify scalable quantum recurrent circuits. These circuits are designed for the efficient simulation of stochastic processes, utilizing the unique recurrent structure of quantum models to address two critical challenges: (1) the training of quantum models, and (2) the distillation of quantum models. Our approach includes the development of hybrid classical-quantum algorithms aimed at capturing the temporal dynamics of stochastic processes and formulating a specific cost function optimized for time-series data analysis. Furthermore, we intend to develop an algorithm dedicated to refining quantum recurrent models through the dynamical decoupling of the system, as opposed to the initial compilation of the quantum operator. This approach is expected to generate quantum circuits with lower memory requirements and reduced circuit depth, effectively mitigating the problem of error accumulation in near-term quantum devices as circuit depth increases. The success of this project promises to broaden the application of quantum algorithms in areas such as machine learning and financial markets, while also narrowing the gap between the theoretical advantages of ideal devices and the real advantages of imperfect devices. This signifies a considerable advancement in our comprehension and employment of quantum computing technologies.
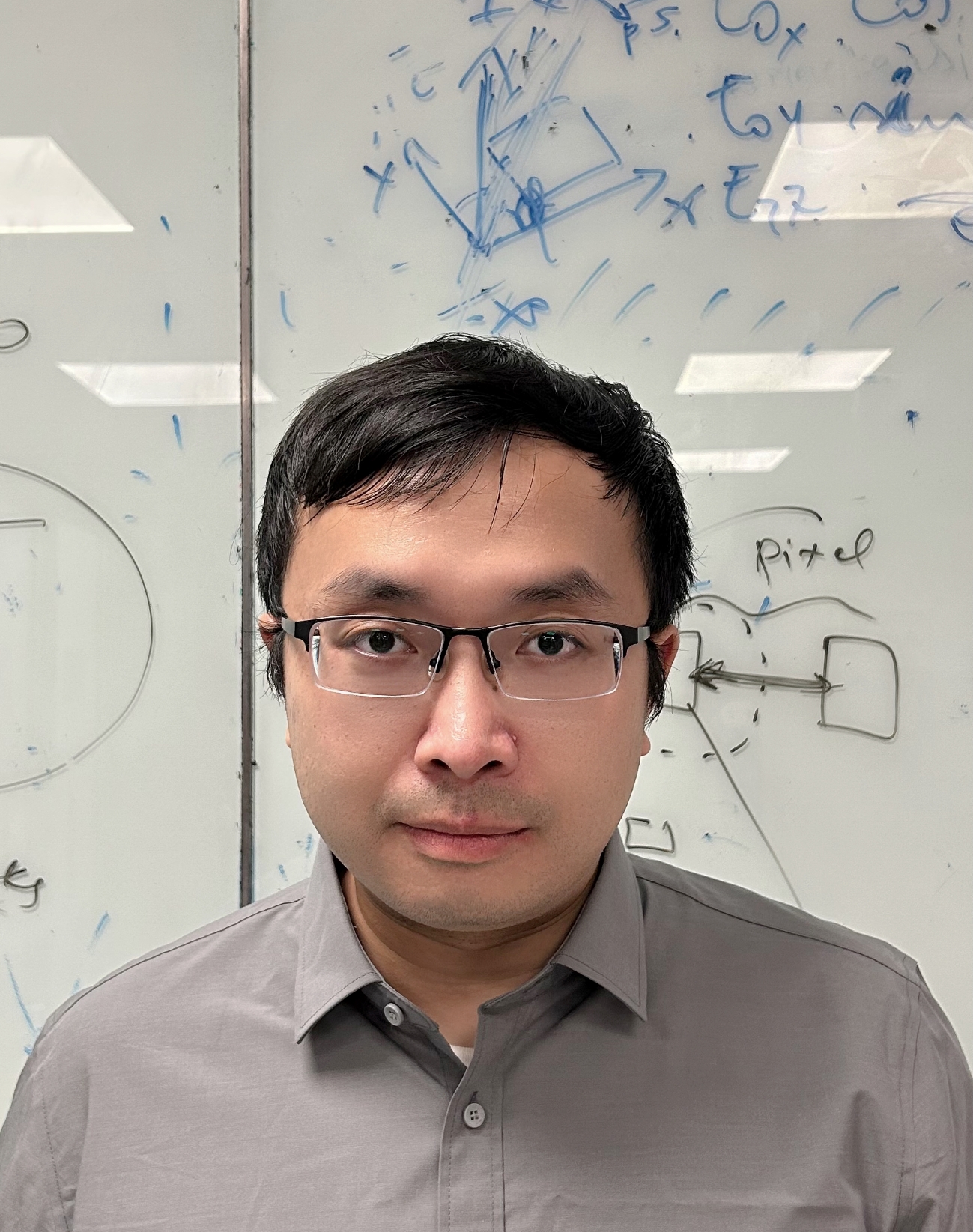
Yang Long
Host School : SPMS
Email: [email protected]
Google Scholar: https://scholar.google.com/citations?user=D_YienkAAAAJ
ORCID: https://orcid.org/0000-0001-7600-3396
Github: https://longyangking.github.io/
Yang Long obtained his Ph.D. in Physics from Tongji University, Shanghai, China in 2020. Currently, he is a research fellow at Nanyang Technological University, Singapore. Dr. Long's research expertise includes investigating spin angular momentum and
new topological phenomena in classical wave systems, directional control of near-field photonics/phononics, and machine learning in fundamental physics. He is currently concentrating on the classification of topological phases of matter without human
knowledge and developing new kinds of unsupervised learning algorithms for replacing complicated abstract mathematics.
- Machine learning in physics
- Topological physics
- Near-field wave physics
- Spin angular momentum in classical waves
Project: Machine learning of topological classifications without human knowledge
Abstract: A significant and unfading theme in fundamental science is the classification
of matter. The classifications of matter reflect the uses and functions of matter. However, the traditional classifications of matter are primarily restricted to using a few common physical characteristics or degrees of freedom, such as elements,
geometrical structures, or chemical compounds. Topological classification, a new classification scheme based on topology, has been gradually introduced into investigations of classifications of matter. Natural matter can be further divided into various
topological classes, such as topological/trivial insulators and topological semimetals, in accordance with topological classifications. Novel topological phenomena between two topologically distinct materials have been observed with plenty of applications
such as topological laser and on-chip THz-region optical transport. Although many theories about topological classifications have been constructed, there are still new topological mechanisms or materials reported very recently. The reason is that
these theoretical approaches heavily rely on abstract mathematics (such as homotopy group, K-theory, or Clifford algebra), which is incomplete, under slow development, and may be incorrect in new cases. Note that many materials previously classified
as “trivial” are found to be topological with new theoretical advances. Therefore, a question is raised: is it possible to dispense with flawed mathematical techniques (e.g., homotopy group and K-theory) or refrain from using any human
knowledge? In other words, can we realize topological classifications only based on raw data collected from matter? In this project, I aim to systematically incorporate machine learning to realize topological classification in a data-driven manner,
in order to abandon the traditional abstract mathematics. The methodology is based on applying unsupervised learning technologies to raw data from Hamiltonian samples to capture topologically distinct phases. In contrast to earlier math-heavy theories,
this data-driven approach will not require any human experience or knowledge. Therefore, it will not miss a “hidden” topological phase due to the incomplete list of topological invariants or theoretical limitations. It also takes practical
constraints (e.g., a finite number of bands) into account, and reveals some previously unnoticed features.

Dao Fuying
Host School : SBS
Email: [email protected]
Personal Webpage: https://daofuying.github.io/
Dao Fuying received a Ph.D in Biomedical Engineering from the University of Electronic Science and Technology of China. Based on a background in biology and computational science, Fuying’s doctoral research focused on origin replication sites identification
in eukaryotic genome through integrative analyses of genome-wide DNA methylation, histone modification profiles, and 3D genomics (Dao et al. 2023, INT J BIOL MACROMOL;
Dao et al. 2022, RESEARCH; Dao et al. 2021a,BRIEF BIOINFORM
(
Highly cited paper); Dao et al. 2021b,BRIEF BIOINFORM (Highly cited paper); Dao et al. 2021c,BRIEF BIOINFORM;
Dao et al. 2019, Bioinformatics (Highly cited and hot paper)). Fuying continued her research
in Dr. Melissa fullwood’s lab as a postdoctoral research fellow, to identify chromatin interactions and cancer biomarkers using artificial intelligence methods.
Research Interests: Dao Fuying is interested in designing and using computational approaches to solve biological issues. Particularly, she prefers to identify chromatin interactions and cancer biomarkers using artificial intelligence methods, aim to develop precise therapies for cancer by identifying specific chromatin interaction-based biomarkers.
Project: Application of deep learning in identifying chromatin interactions and cancer biomarkers
Abstract: In eukaryotes, chromatin is folded into complex 3D structures and dynamically regulates life processes. Use of artificial intelligence methods to identify specific chromatin interaction-based biomarkers enables the differentiation of various cancer subtypes
and the development of precise therapies for more effective cancer treatment. Here I hypothesize that a deep learning model can mine informative features and predict accurately chromatin interactions in RNA-Seq samples. The significance of this work
is that if successful, we will be able to analyze large cohorts of clinical RNA-Seq data for chromatin interactions and suggest potential epigenetic drugs for further drug testing.
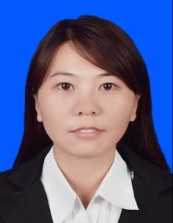
Tian Qingyun
Host School : CEE
Email: [email protected].
Tian Qingyun obtained her B.Eng degree from Southeast University, China in 2016. Then she received her Ph.D. degree from Nanyang Technological University, Singapore, in 2022. After graduation, she worked as a research fellow in Nanyang Technological University and National University of Singapore. Her research mainly focuses on modelling and optimization of transportation system.
Research Interests: Urban transportation, Connected and autonomous vehicles, Infrastructure planning and design, Shared mobility, Intelligent transportation system.
Project: Learning based modeling framework and application in operation management of transportation system
Abstract: In presence of a rapidly increasing urban population and the resultant
travel demand expansion, many major cities in the world are facing the urban challenge of offering efficient mobility services for both passengers and goods. Nowadays, with the development of technology and high-performance computing, the smart
transportation system is being popular in both academia and practice. AI technology based on "learning" from massive amounts of data can identify features and make analysis and predictions more quickly, which provides new ways for transportation
planning, operation, and management. The research project focuses on the application of AI in transportation. The objective is to provide solutions to the operation management of transportation systems. To achieve the goal, AI technology, machine
learning, and transportation model will be required to be integrated into the whole framework.
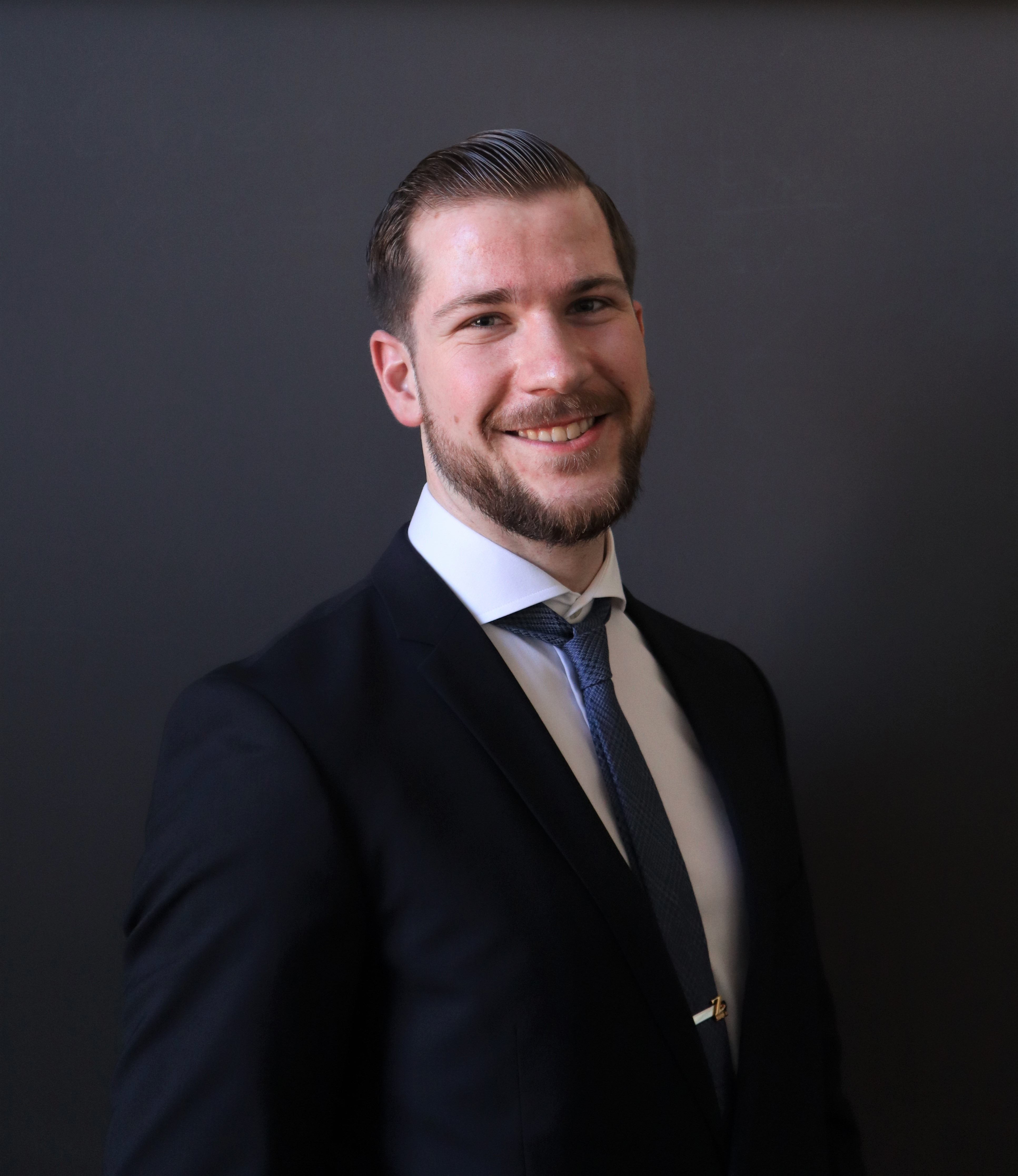
Florian Rossmannek
Host School : SPMS
Email: [email protected]
Personal Website: https://people.math.ethz.ch/~rofloria/
Florian Rossmannek obtained his bachelor's, master's and PhD degree in mathematics from the Swiss Federal Institute of Technology in Zurich in 2018, 2019, and 2023, respectively. During his doctoral studies he was a member of RiskLab Switzerland. Now he is a Schmidt AI in Science Postdoctoral Fellow at Nanyang Technological University Singapore.
Research Interests: approximation and optimization problems in machine learning, neural networks, reservoir computing, applications of machine learning to quantum physics.
Project: Learning Schrödinger’s equation with reservoir systems
Abstract: Machine learning has seen many applications in which it served as a black box to generate
good results. To improve upon this and make the black box interpretable, one needs to understand the application of interest mathematically and tailor the machine learning approach accordingly. In this project, we pursue this methodology for applications
in quantum physics. One difficult challenge therein is to find the so-called ground and excited states, which are energy levels of the system. Knowing these states is fundamental to understanding and being able to simulate chemical reactions. Mathematically
speaking, we design a new interpretable machine learning approach based on reservoir computing to learn eigenvalues of a Hamiltonian operator by considering Schrödinger's equation.
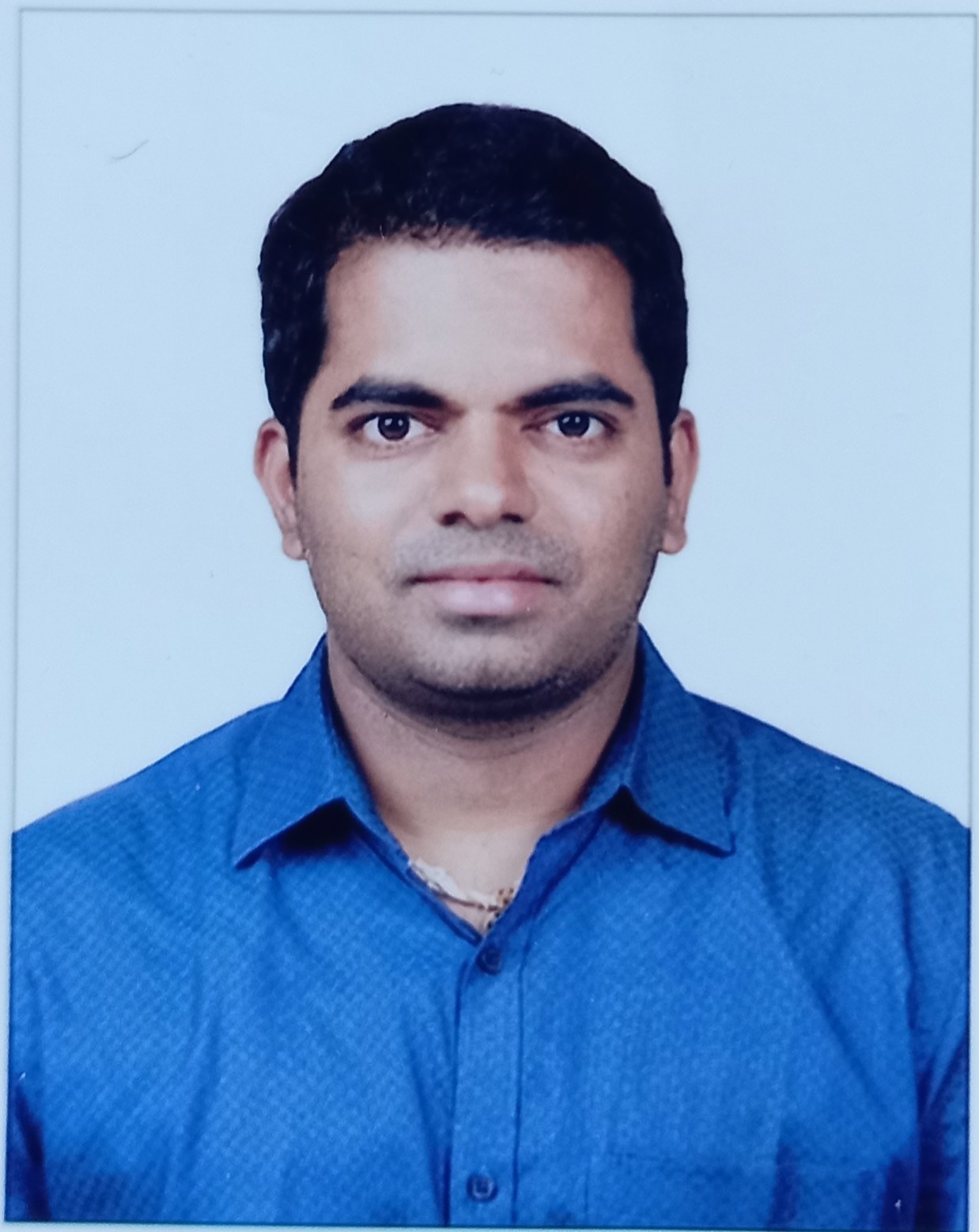
Wadgaonkar Indrajit Pradeepchandra
Host School : SPMS
Email: [email protected]
Indrajit received a PhD in Physics from the Nanyang Technological University (NTU), Singapore in March 2023. During his PhD, he worked on the application of the semi-classical framework (Time Dependent Boltzmann Equation) to decipher ultrafast dynamics
in quantum materials. Before joining NTU, Indrajit completed his master's at the Indian Institute of Technology, Madras, India and then worked as a senior manager for research and development with India's largest automobile manufacturer, Tata Motors
Ltd. During his stint at Tata Motors Ltd. Indrajit spearheaded the computational fluid dynamics analysis at the Advanced Engineering department wherein he worked primarily on fuel-cell technology for the future. Indrajit is a recipient of the Government
of India Scholarship for higher studies, the J.N.Tata Endowment Scholarship (2018), Nanyang Research Scholarship (2018), the first Imperial-TUM-NTU Global Fellows Programme(2020) and The Eric and Wendy Schmidt AI in Science Postdoctoral Fellowship
(2023).
Research Interests:
Ultrafast dynamics in quantum materials, semiclassical analysis of quantum systems, theoretical quantum physics and statistical mechanics
Project: Artificial Intelligence assisted state of the art modelling of phonon Poiseuille flow in graphene and thin graphite
Abstract: Clock speed and hence processor speed for modern electronics has been stagnant for the last two decades owing to restrictions on the maximum rate of heat dissipation, which in turn is a result of the limited thermal conductivity of component materials.
In principle, hydrodynamic Poiseuille flow of phonons in graphene promises an infinite thermal conductivity which can be potentially engineered to realise revolutionary technological applications in modern electronics. However, the inherent complexity
in describing this phenomenon has so-far thwarted the development of real-world applications by preventing a satisfactory numerical model which can augment the on-going experimental advances. Although the Time Dependent Boltzmann Equation (TDBE) is
a widely acknowledged framework to model hydrodynamic transport in materials, the super-linear scaling with precision N for the numerical solution of its scattering integral term presents a formidable challenge and this has greatly restricted the
applicability of the TDBE. Over the last few years, we have developed a novel solver for the solution of this very term, which greatly mitigates the scaling challenge. For instance, for a 3-leg phonon scattering description our solver shows a scaling
with precision as N^2.5 in contrast to the N^4 scaling of conventional methods. This significant gain has already enabled us to successfully use the Boltzmann approach to decipher far-from-equilibrium ultrafast dynamics in upcoming quantum materials
like CNTs and GeTe. In this project I propose to augment our solver with i) An Artificial Intelligence (AI) predictor of the morphology of the complex hypersurface involved in the scattering integrals of the TDBE and ii) an AI-assisted quadrature
method. With these enhancements I envisage a further decrease in the scaling of the numerical cost to N^2 leading to a significant gain even at moderate precision, thereby allowing a detailed description of hydrodynamic phonon flow in graphene under
a temperature gradient and even for complex geometries. The success of this project will not only result in a first-ever modelling of phonon Poiseuille flow in graphene and thin graphite but will also open exciting avenues for major technological
advances through the realisation of ultra-high heat dissipators for modern electronics.