Math for AI: Can Mathematics provide a Solid Foundation for AI? by Assoc Prof Xia Kelin
This Discovery Science Seminar, organised by IAS NTU in collaboration with the Graduate Students’ Clubs of SPMS and CCDS, featured an insightful talk by Assoc Prof Xia Kelin from NTU, titled "Math for AI: Can Mathematics Provide a Solid Foundation for AI?" The talk explored the role of advanced mathematics in artificial intelligence (AI), with a particular focus on its applications in molecular sciences.
Prof Xia’s research focuses on Mathematical AI in molecular sciences. He obtained his PhD from the Chinese Academy of Sciences and later served as a visiting scholar at the Department of Mathematics at Michigan State University from 2009 to 2012, where he subsequently worked as a visiting assistant professor. He joined NTU in 2016 and was promoted to associate professor in 2023. Prof Xia has published over 80 papers in prestigious journals, including SIAM Review, Science Advances, npj Computational Materials, and ACS Nano.
Prof Xia’s seminar provided a broad and comprehensive overview of advanced mathematics-based data representations and featurisation for molecular sciences. He began by highlighting the rapid advancements in experimental data and computational power over the past century, which have significantly propelled the development of AI. However, he pointed out a major challenge in developing AI-based learning models: efficient data representation and featurisation. This challenge is particularly relevant in chemical and biological sciences, where the relationship between a molecule's structure and its chemical and physical properties is well-established.

Insightful presentation by Prof Xia on Mathematical AI in molecular sciences.
Prof Xia introduced the audience to persistent homology, a widely recognised method in topological data analysis (TDA). Persistent homology represents data as a series of simplicial complexes, known as a filtration, and computes topological features, such as Betti numbers, on this filtration. These topological features allow for the incorporation of higher-order and multiscale information in the data. To demonstrate this concept, Prof Xia used simple molecular structures, such as fullerene, and showed how these multiscale topological descriptors could be combined with machine learning models, like random forests, to predict the chemical, physical, and biological properties of molecules.
The seminar then transitioned to more advanced topics, where Prof Xia discussed topological and geometric deep learning models based on message-passing graph neural networks. These end-to-end models can automatically learn the appropriate representation of a molecule and predict its properties, making them particularly powerful for analysing large molecular datasets. Prof Xia also demonstrated the utility of algebraic descriptors such as persistent Tor algebra for protein-protein interaction analysis. Finally, he introduced QCFormer, a transformer model based on quotient complexes that encodes periodic information for material property prediction.

Attendees actively participating in the Q&A session.
Prof Xia’s talk resonated with a diverse audience, attracting questions from students and researchers across multiple disciplines. Mathematics students posed inquiries about the intricate details of using algebraic topology for data representation, seeking to understand the deeper mathematical foundations behind these techniques. Meanwhile, computer science students showed great interest in the application of mathematical frameworks in designing and optimising deep learning models. The engaging discussion that followed underscored the broad relevance of Prof Xia’s work across different fields of study.
Prof Xia's seminar successfully illuminated the vital role that advanced mathematics plays in the development of AI models, particularly in the molecular sciences. By showcasing the power of topological, geometric, and algebraic approaches, he provided the audience with valuable insights into how mathematical frameworks can revolutionise data representation and learning. The seminar not only deepened the participants' understanding of these concepts but also sparked curiosity about the future intersections of mathematics and AI.
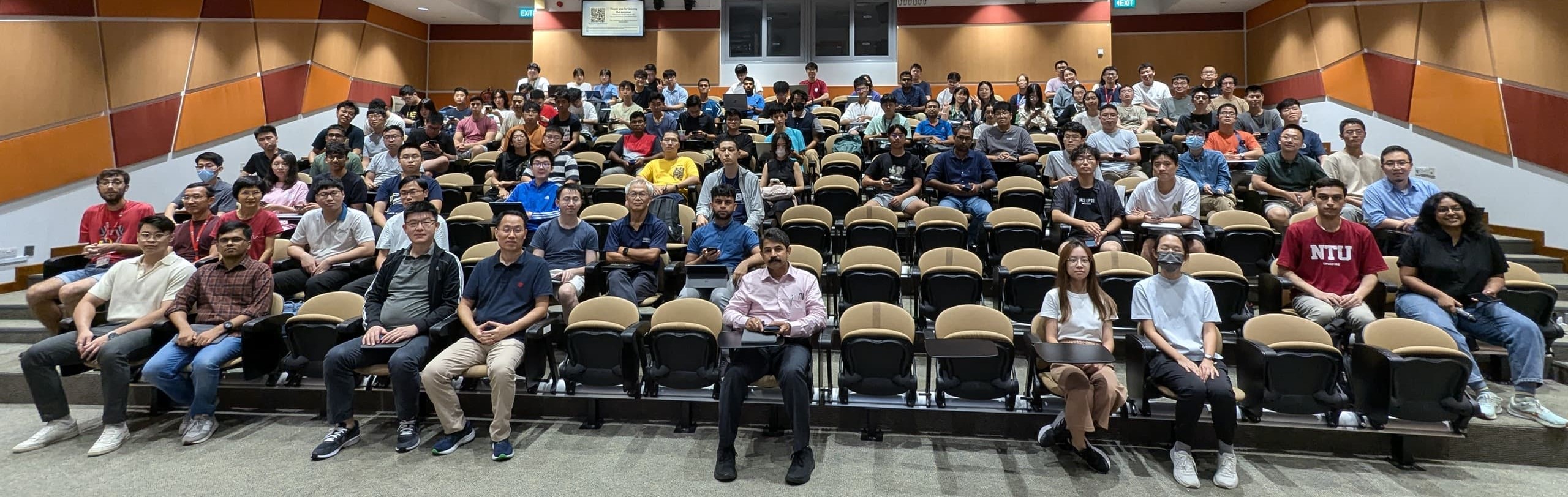
Written by: Yasharth Yadav | NTU School of Physical and Mathematical Sciences Graduate Students' Club
"The relation between math and feature engineering is insightful and thought provoking." - Tang Jingxiang (PhD Student, SPMS)
"Different insight of data representations!" - Yan Mingxue- (PhD Student, IGP-NEWRI)
"It is a very in-depth and wide field of knowledge."- Yeo Feng Ren (Undergraduate, SCSE)
Watch the video recording.
